Should AI Analyse Patient Genetic Data?
Should AI Analyse Patient Genetic Data? Maybe the Public Has the Answer
By Jack Harrison,
Master of Science student, The University of Melbourne
Artificial intelligence (AI) is a hot button issue for society. No longer confined to science fiction or data science, AI is now used everywhere from business to poorly written student essays. But what does it mean for medicine? And more specifically, what can it do for genomic medicine?
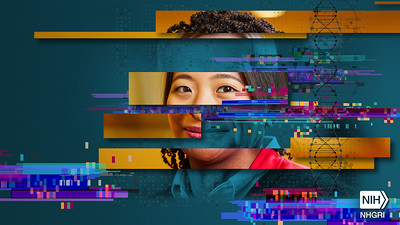
Personalised medicine
Genomic medicine refers to how a person’s genes influence their health and their responses to medical treatments. By tailoring a person’s treatments to their unique genetic make-up, we can personalise their medical care for a better outcome.
AI has the potential to revolutionise genomic analysis and get more informative results to patients faster than ever.1 However, with the growing use of ‘whole exome sequencing’ – sequencing all of an individual’s genes – the amount of data being generated is too great for existing IT infrastructure to handle.2
Automated tools are already being used in the genetic diagnostic setting, but the use of AI has new considerations. Public perspectives are imperative to ensuring the safe and equitable use of AI. Any medical field that wants to effectively incorporate AI needs to meaningfully engage with the public.
Public engagement
To learn more about public perspectives on AI in healthcare, we ran focus groups with Australians from all walks of life. By engaging a diverse range of participants in our focus groups, we were able to hear a more diverse range of perspectives on genomic AI.
During our sessions, participants were asked how they felt about having their own genetic data analysed by AI, as well as their thoughts on data security, and consenting to use of AI in the analysis process.
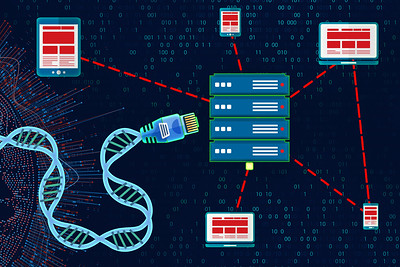
How are we feeling?
Pretty good actually! Polls from participants showed that the vast majority were comfortable with their own DNA being analysed by AI. In general, participants became even more comfortable with AI analysis after group discussions, which corroborates research on AI in other medical fields.4
There seems to be a general level of trust among the public, and this trust can be improved through education and open discourse about AI in medicine. Reasons given for trusting AI ranged from the potential benefits, like reduced risks associated with disease, to preferring AI analysis. Many participants related this to previous negative experiences with healthcare professionals.
But it’s not all sunshine and rainbows. Most participants agreed that there should be some sort of human checking mechanism, and, when pressed further, many still expressed some level of distrust. This distrust wasn’t always linked to the technology itself, but a general feeling of distrust about computer analysis. For others, they considered genomic data to be more personal or far-reaching than other medical data, and were less comfortable with the use of AI to analyse it.
Overall, participants strongly agreed on the potential benefits of AI in genomic medicine. They talked about how it could reduce wait times for patients, lead to new discoveries, reduce the workload for researchers and reduce bias and errors in analysis.
Great! Time to steamroll ahead?
Not quite. In line with previous research, participants found that the potential effect of AI on error was a double-edged sword.5
AI has the potential to reduce human error, and lead to more accurate diagnoses for patients, but it also has the potential to introduce and reinforce bias against marginalised and underrepresented groups. This is a problem that we already see with AI in public discourse, such as with image generation unintentionally reaffirming racial and gender biases.6
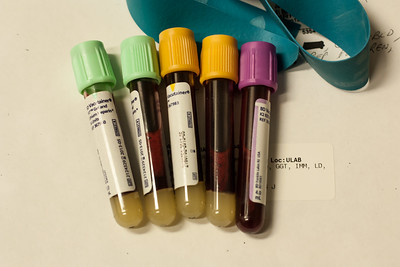
While most participants were comfortable with AI analysing their data, they also strongly preferred to have a human professional check the results. Participants from marginalised backgrounds were also concerned that genomic AI tools could be used against them to justify discrimination.
These are just some of the potential issues that AI could introduce.
What happens when things do go wrong?
In short, there’s no clear answer. But that’s just another reason why engaging with the public is so important.
Like with any technology, if something goes wrong, there is a discussion to be had about who’s at fault and who should be held accountable. If the doctor gives you inaccurate or incorrect results, are they at fault? Or is it the fault of the technology for giving the doctor those results in the first place?
AI may further muddy this discussion. Machine learning is a process through which an AI learns from its training data. It involves an AI model learning from existing data to make generalisations about unseen data, and adjusting based on any new information.7 This allows for the creation of far more powerful tools than anything that could be coded manually.
Unfortunately, this process can make it difficult – or even impossible – to figure out how or why the AI model produces results.8
If an AI model produces a result that is outside of our current understanding of genomic medicine, but not necessarily in violation of it, can we trust it? Perhaps it can piece together information in a way that no human has – yet. Furthermore, should such results be shared with patients by medical professionals?
Focus group participants who were presented this scenario didn’t always have a clear answer. For many participants, this generally reaffirmed the need for human oversight of these tools.
Clearly, this is a complicated space. More research is needed into the thoughts and preferences of the public and professionals alike. Guidelines also need to be developed for the building and use of AI.
Jack Harrison is completing a Master of Science in Genomics and Health at The University of Melbourne and Murdoch Children’s Research Institute.
References:
- O’Brien, T. D., et al. (2022). Artificial intelligence (AI)-assisted exome reanalysis greatly aids in the identification of new positive cases and reduces analysis time in a clinical diagnostic laboratory. Genetics in Medicine, 24(1), 192–200. doi.org/10.1016/j.gim.2021.09.007
- Best, S., et al. (2024). Reanalysis of genomic data in rare disease: current practice and attitudes among Australian clinical and laboratory genetics services. European Journal of Human Genetics. doi.org/10.1038/s41431-024-01633-8
- Vears, D. F., & Gillam, L. (2022). Inductive Content analysis: a Guide for Beginning Qualitative Researchers. Focus on Health Professional Education: A Multi-Professional Journal, 23(1), 111–127. doi.org/10.11157/fohpe.v23i1.544
- McCradden, M. D., et al. (2020). Conditionally positive: a qualitative study of public perceptions about using health data for artificial intelligence research. BMJ Open, 10(10), e039798. doi.org/10.1136/bmjopen-2020-039798
- Wu, C., et al. (2023). Public perceptions on the application of artificial intelligence in healthcare: a qualitative meta-synthesis. BMJ Open, 13(1), e066322. doi.org/10.1136/bmjopen-2022-066322
- Fernández, A., & Garrido-Merchán, E. C. (2024). A Taxonomy of the Biases of the Images created by Generative Artificial Intelligence. ArXiv (Cornell University). doi.org/10.48550/arxiv.2407.01556
- Janiesch, C., et al. (2021). Machine learning and deep learning. Electronic Markets, 31(31), 685–695. Springer. doi.org/10.1007/s12525-021-00475-2
- Wadden, J. J. (2021). Defining the undefinable: the black box problem in healthcare artificial intelligence. Journal of Medical Ethics, 48(10), medethics-2021-107529. doi.org/10.1136/medethics-2021-107529