Problems and Problem Solving Around Epilepsy
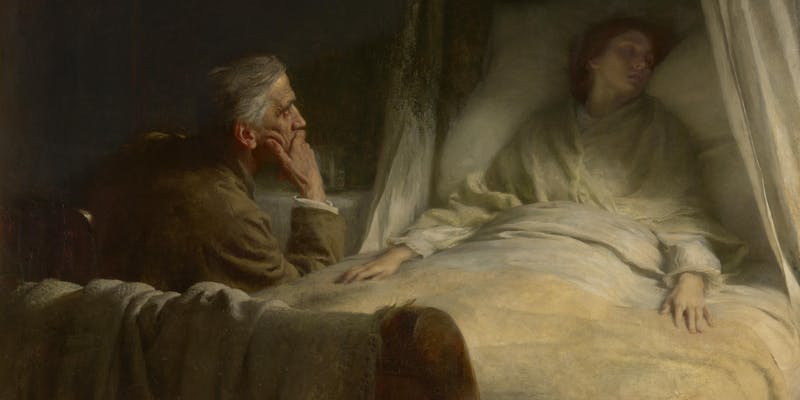
by Catriona Nguyen-Robertson MRSV
This article follows a presentation to the Royal Society of Victoria on 28 of November, 2019 titled “Epilepsy, Algorithms and AI: Personalised Seizure Forecasting” by Professor David Grayden.
“Epilepsy is a big problem,” according to Professor David Grayden, a biomedical engineer based at the University of Melbourne. It affects 1% of the world’s population.
Among epileptic patients, 70% are effectively treated with drugs and live a generally normal life, but the other 30% are resistant to these drugs. Some can get around this by having invasive surgical procedures. Yet despite progress of the last 30 years to improve epilepsy treatment, the percentage of patients who cannot be treated remains at 30%.
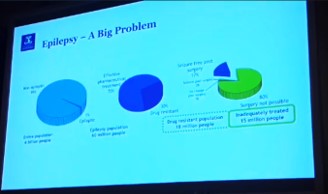
Seizures – whether big or small – can make it dangerous for people going about their everyday lives. If you do not know when a seizure is coming, it limits the amount of control you feel you have over your body. For those patients who cannot control their seizures with drugs, swimming, bathing, driving, and certain jobs become too dangerous. If they lose their job, this leads to stigma and social isolation. But above all, the most pressing reasons advance epilepsy treatment is the prevention of sudden death due to seizure.
One of the most debilitating aspects of epilepsy is the uncertainty of when a seizure will occur – even if they are as infrequent as two a year. If you don’t know when the seizures will come, you cannot know when you are safe.
Professor Grayden wants to remove this unpredictability in a person’s life. The ability to predict the onset of seizures would change the lives of over 15 million people.
The Melbourne NeuroVista Seizure Prediction Trial was launched in 2009 as a collaboration between multiple institutions, including the Graeme Clark Institute. In the trial, 16 electrodes were implanted in the brain to measure brain activity, which communicated with an external seizure warning device. A total of 15 patients with drug-resistant epilepsy were enrolled in the study and monitored continuously for up to three years – the first time a study like this had ever been done.
An algorithm was trained using data from each patient’s electroencephalogram (EEG) (their electrical brain activity), and for most patients, was able to indicate low, moderate or high probability of seizure occurrence with a series of coloured light indicators. A red light indicated high probability of seizure – and seizures did often occur at times that the light was red. This demonstrated that seizure prediction was indeed possible, but the red light seemed to be on for most of the time, which was not ideal.
It is difficult to train algorithms on few seizures, so the team needed to apply more sophisticated artificial intelligence (AI) approaches. In partnership with IBM Research, they generated a deep learning neural network, the “TrueNorth chip”, a cognitive computer that combines AI and machine-learning algorithms to mimic the behaviour of the human brain. Team input previously recorded intercranial EEG recordings from the original Neurovista study participants for the deep learning system to develop a new warning model. The deep learning systems continually adjusted itself as it made mistakes (much like humans do). Its caveat, however, was that it relied on pre-existing training data. An even better algorithm was needed so that each individual could have a personalised warning system.
Data scientists around the world were invited to develop an algorithm for the team’s data and over 10,000 were submitted that aimed to improve the sensitivity of the epilepsy monitor – making sure that the device gets its warnings right without having the red light constantly on. The team selected the top several algorithms that had the best balance between the two – the next problem was that most of the algorithms required a super-computer to run.
The solution was to consider the brain’s capacity to rapidly change state. Prior to a seizure, brain signals can exhibit a critical slowing, which indicates that the brain is wobbling between normal and seizure states. The team analysed EEG recordings to find key signatures of critical slowing prior to seizures, which turned out to be the most reliable indicator for their seizure forecasting algorithms.
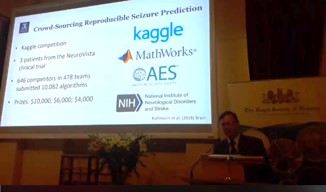
Seizure diaries can also be used to forecast seizures so long as patients accurately monitor their own seizures. The team observed cycles in which people tended to have seizures at particular times of the day, or week, or month (e.g. if a person has a seizure once a week, it tends to be on a weekend). Tracking these cycles could hence help determine times a person is most at risk or not at risk at all.
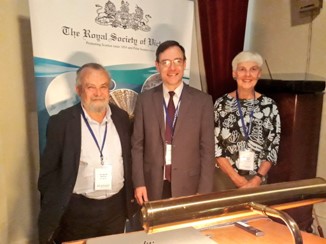
Engineering, biology, bioinformatics, and data science have converged to make it possible to forecast seizures. Professor Grayden and colleagues are working to ensure that each person will be at low risk more than 50% of the time so that they can go about their everyday lives without the worry and stigma associated with unpredictable seizures.