What Can Brain Cells on a Microchip Tell Us About Intelligence?
by Dr Brett J. Kagan, Cortical Labs
- What is intelligence?
- How can we create an intelligent system?
The former question has remained contentious since it was first posed, with disagreements even as to the definition of the original Latin, intelligentia, (1, 2), while the latter has inspired countless science fiction stories. Despite fragmented semantic use and fantastical fiction, exciting advancements in STEM offer pathways to empirically investigate – and perhaps one day answer – both questions.
While substantial research has been devoted to the development of artificial intelligence through silicon computing hardware (3, 4), other work has focused on new hardware approaches such as “neuromorphic engineering” – using electrical circuits to mimic the way our nerves function(5).
Whether “intelligence” can be created within hardware is a fascinating question, and is one that remains unanswered despite significant efforts. In contrast, biological intelligence from a neural source offers a “ground truth” of opportunity: from flies, to cats, to humans, all animals display a degree of generalised intelligence. As such, the question becomes not ‘if’ general intelligence can arise from something artificial, but ‘how’.
Our recent work (6) offers one approach for how biological intelligence could be synthesised, which we have termed Synthetic Biological Intelligence (SBI).
A Shared Language of Silicon and Biology
Neuronal networks use an electrical signal to transfer information from one cell to another. This allows us to use electrical impulses as a ‘shared language’ to bridge the gap between traditional computing hardware and neurons cultured in a Petri dish.
Multielectrode arrays (MEA) have been used since the 1990s as a way to both measure electrical activity from neural cells, or to deliver an electrical signal to neural cells on a microchip (7).
Using this setup, neural networks can be observed both without stimulation, or in response to it, in what is known as an ‘open loop’ system. In this set up, the cells respond to the stimulation, but the activity does not impact future incoming information.
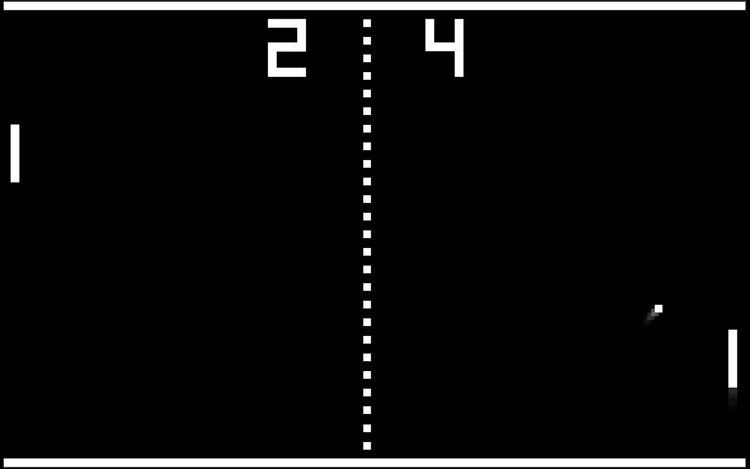
A system where we could ‘close the loop’ and have the activity from the cells impact an environment leads us to the question: can we ‘teach’ these networks to act in a certain way? Can we ‘teach’ them to anticipate a stimulus that hasn’t arrived yet, or to produce a new, modified response? This would allow us to build a bridge between the electrical activity in neural systems and that used in computing systems.
In our recent work (6), we implemented a real-time closed-loop system to simulate a simplified version of the classic arcade game, ‘Pong’ (Figure 1).
To do this, we delivered electrical stimulation into cultures of neural cells. Our setup ‘told’ the neural cells the x and y positions of the ball relative to the paddle, using the ‘location of the stimulation’ and the ‘rate of stimulation’. In response to this stimulation, the cells produced electrical activity, which was ‘read’ by the detector and used to move the paddle.
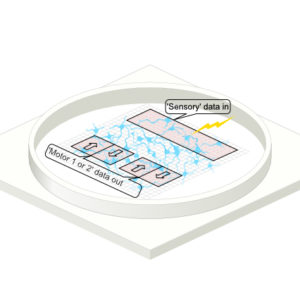
When the paddle missed the ball, the game would reset, with the ball reappearing at a random point. For the neural cells, this meant that missing the ball required greater energy usage to respond to this new, unpredictable location.
This setup allowed the outgoing activity to change incoming information dynamically, i.e., it was able to move the virtual paddle. In this instance, the neuronal cells on a synthetic chip appeared to have become goal-directed– a step closer to intelligence.
Guiding Principles of Biological Intelligence
Building a bridge between electrical signals and neuronal activity alone is not sufficient. We still need to figure out how to motivate neurons in a dish to act in any specific way, especially to achieve a goal or show an ‘intelligent’ response. While we, or other animals, often are predisposed to act in a particular way or do one activity over another, for cells in a dish, there is nothing obvious to act as a guide. Neural cultures in a dish are unlikely to have naturally occurring reward pathways present in animals or humans, like the hit of dopamine we get from pleasurable experiences. Even if they do, there is no special way to “reward” or “punish” these cells in real-time (as is done with machine learning algorithms) with any degree of reproducible control.
Instead, we first need to identify how intelligence arises at the most basic level, by looking at a theory called the ‘Free Energy Principle’. Simply put, this theory suggests that all living systems which interact with external environments, from cells to humans, are trying to do something called ‘free energy minimisation’ – trying to reduce uncertainty or minimise surprising information from the environment. When we receive visual (sensory) information, we can make an internal model of our environment in our brain, e.g., of a glass of water on a table. By reaching over to pick up this glass of water, we can test whether this ‘internal model’ matches the external world. If it does, we’ll be able to pick up the glass. If the models do not match, we might unintentionally and unexpectedly knock the glass over (see Figure 3).
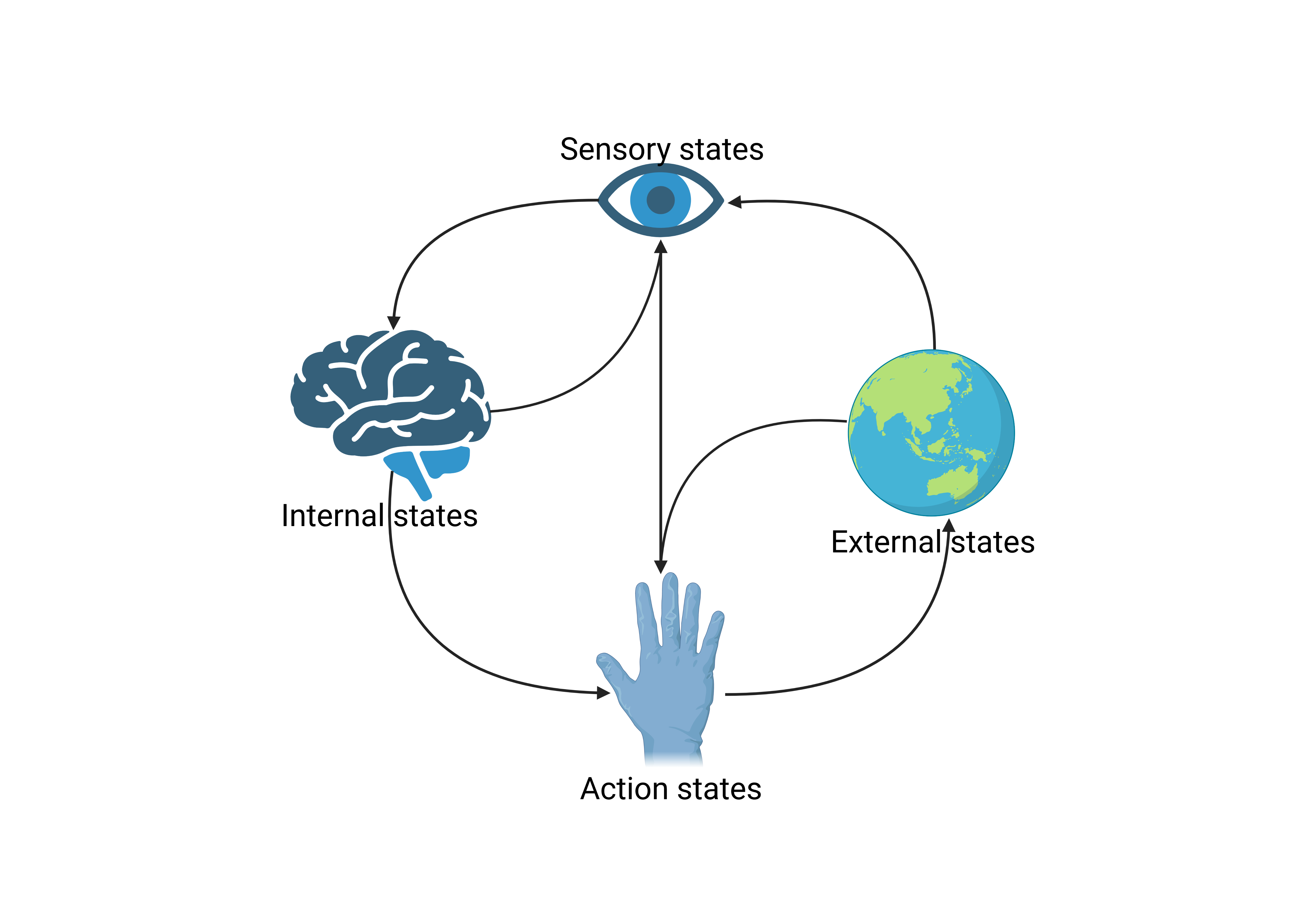
It makes sense that prediction is important; it would be impossible to survive in a changing world without it. Improving this prediction can be done in two ways, either by predicting the environment better, or by making the environment more predictable.
While the neurons in a dish used in our work are far too simple a system to do anything as complex as thinking about a glass of water, under the Free Energy Principle they should still try to minimise uncertainty or surprise from environmental information (via electrical stimulation).
For our game of Pong, we provided a random feedback stimulation (a ‘negative’ response) in response to any activity that resulted in the ball being missed, and a predictable stimulation (a ‘positive’ response) following the paddle hitting the ball. The result was that the neural cells appeared to self-organise their activity and got better at playing the game, supporting this as at least one driver behind intelligent behaviour.
Applications and Opportunities: Basic Science
Intelligence is a fascinating concept, especially in the context of that generated by a human brain. This is best summarised in the now famous quote by Pugh (circa 1983):
“If the human brain were so simple
That we could understand it,
We would be so simple
That we couldn’t.”
What we are hoping for is that SBI can offer the research community a simple model to help us understand intelligence. Of course, trying to understand how intelligence arises using animals or humans is very difficult as both have multiple layers of compensatory mechanisms; trying to disrupt or isolate one mechanism alone is almost impossible. Yet using SBI offers a simplified approach. For example, we recently identified interesting changes in the patterns of electrical activity in neural cells when they were presented with different types of information in response to an action (6). While we previously explored the Free Energy Principle in our recent work (6), this is just one idea for why biological intelligence acts as it does. Other imperatives apart from free energy minimisation also likely exist and other ways that information can be organised in neural systems (8).
Applications and Opportunities: Translational Research
SBI may also prove useful in researching how we can understand or treat illnesses, through preclinical drug discovery and cell-based disease modelling.
Neural systems like the brain are very challenging to model, as we need to recreate the key functions of this complex organ. The brain’s core function is to receive and then process information, before responding in an adaptive way, and current cell models used for drug discovery or disease modelling usually do not provide any structured stimulation to the cells in the form of an electrical interface.
By providing simple neural systems with the chance to process structured information and alter activity in meaningful and measurable ways (like the Pong example), it provides a chance to better understand how a drug or disease may impact more complicated systems such as our brains.
Finally, being able to generate cells from different genetic backgrounds with induced pluripotent stem cell technology also opens the opportunity to explore this in a personalised approach to see how different genetic backgrounds respond. In this way, SBI technology can be very useful in translational research for neurological focuses.
Applications and Opportunities: Into the Future
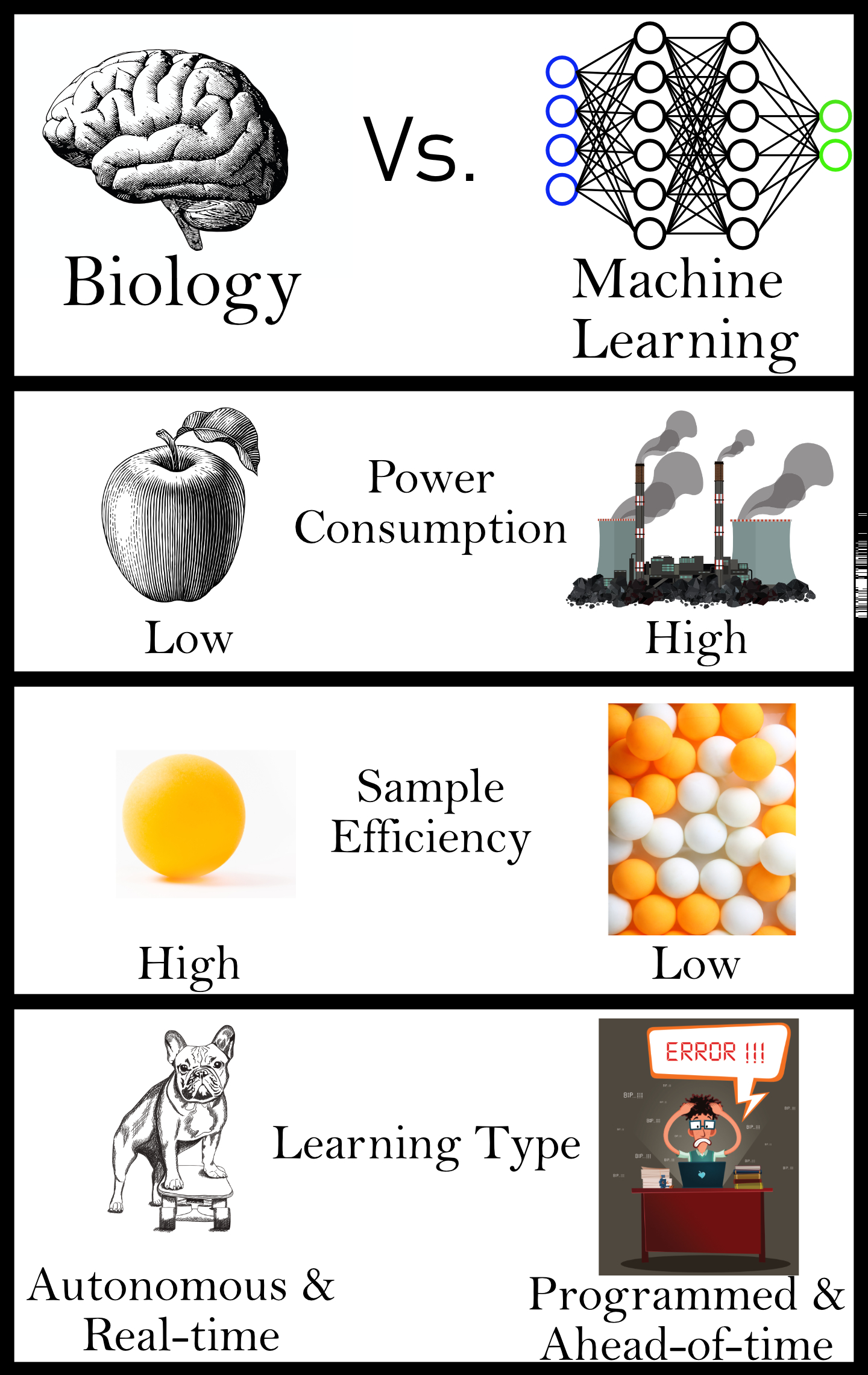
While there is a clear need to make sure this research is developed in an ethically responsible way (10), there are reasons to be excited by SBI’s potential, particularly when compared to current machine learning (summarised in Figure 4).
Maximising the potential of SBI could provide access to a real-time learning system able to display generalised intelligence and function with relatively low power consumption.
Current machine learning algorithms require enormous data to converge on ideal solutions, yet in contrast biological intelligence is capable of rapid highly sample efficient learning(11). Likewise, although humans and animals display considerable flexibility in their behaviours yet are able to pursue goal-directed activities despite massive changes in the environment or information quality, machine learning remains highly fragile and prone to failures when even minor changes are introduced (12).
Finally, running machine learning algorithms currently requires considerable power, using potentially millions of watts (13), while biological neural systems can operate in essentially glorified sugar water(14). Indeed, the power consumption requirements from current machine learning methods are so great as to preclude consumer-wide access to this technology in a personal capacity (without massive changes to either power generation or consumption technologies)(15).
If biologically-based intelligence could be leveraged, it may offer the possibility of a thermodynamically sustainable system, accessible at a personal level, that would act in a simple, efficient and adaptive manner. Such intelligence does not need to equal or surpass a human to be immensely valuable.
Harnessing even basic levels of biological intelligence could have numerous compelling applications. Perhaps it may even help answer the questions of: what is intelligence and how can we create a generally intelligent system?
Acknowledgements
Sincere appreciation to Professor David Walker and Mr Scott Reddiex for their assistance with this article. Some images were produced through Biorender.
References
- P. H. Schonemann, in Encyclopedia of Social Measurement, K. Kempf-Leonard, Ed. (Elsevier, New York, 2005; https://www.sciencedirect.com/science/article/pii/B0123693985005247), pp. 193–201.
- W. C. M. Resing, in Encyclopedia of Social Measurement, K. Kempf-Leonard, Ed. (Elsevier, New York, 2005; https://www.sciencedirect.com/science/article/pii/B0123693985000839), pp. 307–315.
- D. Hassabis, D. Kumaran, C. Summerfield, M. Botvinick, Neuron. 95, 245–258 (2017).
- B. M. Lake, T. D. Ullman, J. B. Tenenbaum, S. J. Gershman, Behav Brain Sci. 40, e253 (2017).
- S. Kumar, R. S. Williams, Z. Wang, Nature. 585, 518–523 (2020).
- B. J. Kagan et al., Neuron, S0896627322008066 (2022).
- Y. Jimbo, H. P. C. Robinson, A. Kawana, IEEE Trans. Biomed. Eng. 45, 1297–1304 (1998).
- F. Habibollahi, B. J. Kagan, D. Duc, A. N. Burkitt, C. French, bioRxiv (2022).
- A. Attinger, B. Wang, G. B. Keller, Cell. 169, 1291-1302.e14 (2017).
- B. J. Kagan, D. Duc, I. Stevens, F. Gilbert, AJOB Neuroscience. 13, 114–117 (2022).
- F. Habibollahi, A. Gaurav, M. Khajehnejad, B. J. Kagan, Accepted NeurIPS 2022, 16.
- L. Fan, P. W. Glynn, The Fragility of Optimized Bandit Algorithms (2022), , doi:10.48550/arXiv.2109.13595.
- N. P. Jouppi et al., Commun. ACM. 63, 67–78 (2020).
- W. B. Levy, V. G. Calvert, Proc. Natl. Acad. Sci. U.S.A. 118, e2008173118 (2021).
- C. Freitag et al., Patterns. 2, 100340 (2021).