Welcome to the Fold
Welcome to the Fold: The Nobel Prize-winning AI driving scientific discovery
By Breana Galea,
Master of Biomedical Science Student, The University of Melbourne
Imagine if you could use a sequence of letters to unlock the secrets of treating incurable diseases, conserving the environment, and countering antibiotic resistance. This may sound like science fiction, but a new artificial intelligence (AI) program is making it a reality.
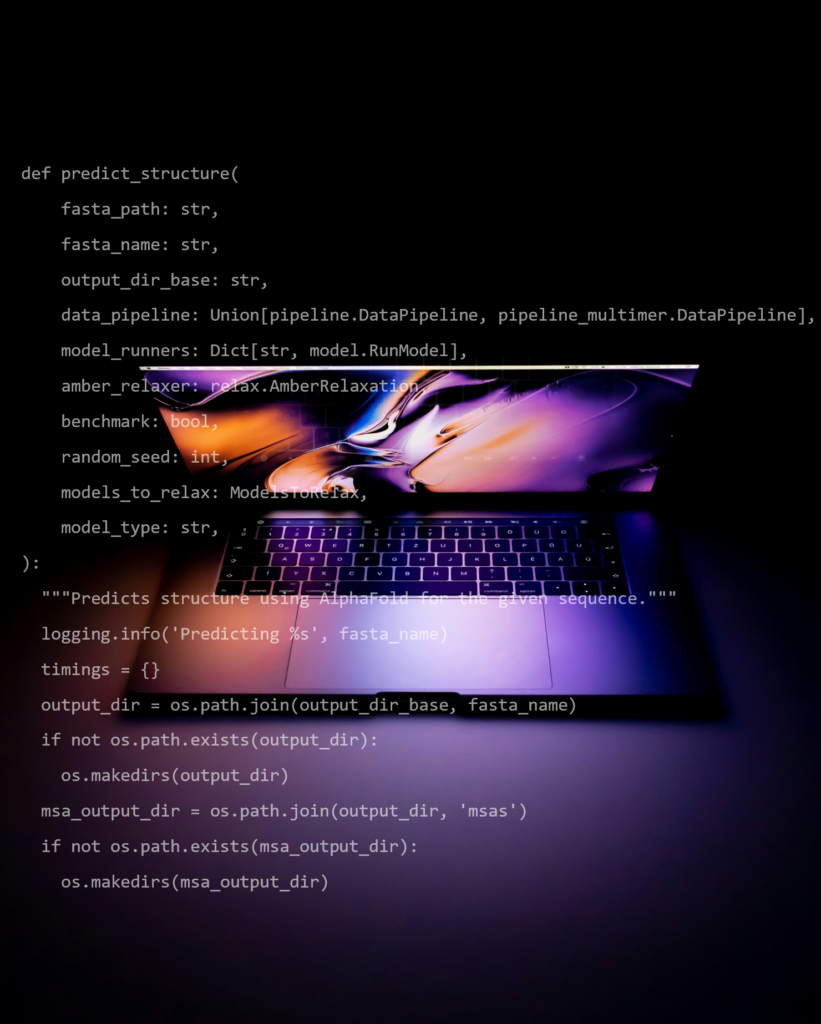
For over 50 years scientists contended with the “protein folding problem”: while DNA tells us the order of the amino acids in a protein, it’s much harder to predict a protein’s three-dimensional shape.1 As a protein’s structure is critical for its function, predicting a protein’s shape is important for understanding its production and interactions.
In 2020, the AI program ‘AlphaFold’ was released. This program can predict the structures of proteins with high accuracy,2 and its arrival is a potential revolution in various fields of research.
Nobel Prize? No surprise
Last year, the Nobel Prize in Chemistry was awarded to the creators of AlphaFold, Sir Demis Hassabis and Dr John Jumper at Google DeepMind, alongside Professor David Baker.3 To many, this did not come as a surprise, as the significance of AlphaFold has been clear since its release. The technology is highly effective, freely accessible, saves significant resources, and is applicable to a wide range of scientific fields.
From drug discovery to the breakdown of plastic pollution, AlphaFold has made its mark. The AI program is designed to solve protein structures, but what does this mean and why is it important?
Life’s machines called proteins
Proteins are involved in most natural and many industrial processes, from functions of the immune system to harnessing nature to break down plastic pollution. They consist of building blocks called amino acids, which can be visualised as a string of different beads forming three-dimensional shapes.
A protein’s structure determines how it functions. Learning about the detailed structure of a protein is regarded as “solving” its structure, as this provides many clues that help understand its role, how it interacts with other molecules, and how we can manipulate it.
Scientists have dedicated decades to solving protein structures, allowing for the development of valuable new technologies, such as vaccines for COVID-19. Many would recall seeing the SARS-CoV-2 virus in the media: the surface of the virus dotted with spike proteins. Establishing the structure of this spike protein revealed key regions that could be targets for vaccine development.4,5
Traditional techniques have limits
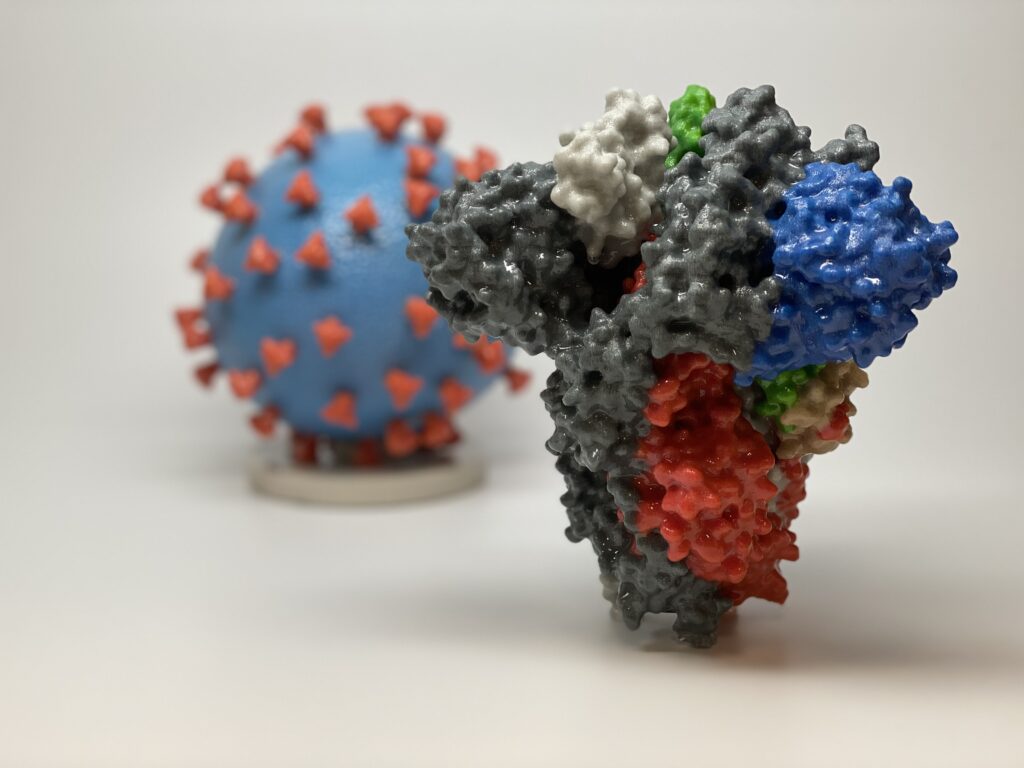
Experimental methods for determining protein structure have existed for over half a century, enabling scientists to dip into the protein structure pool. The results of approximately 200,000 protein structures have been collated in an online database named the Protein Data Bank (PDB).6 However, the number of proteins in the world is huge – in the billions. The techniques used to understand each individual protein can be time-consuming and resource-intensive, requiring intricate multi-step processes and years of expertise.
Other tools are needed to streamline this experimentation, speeding up our discovery and understanding of protein structures. This is where AlphaFold comes in.
Paradigm-shifting predictions
The potential of the AI program AlphaFold was first realised at the fourteenth international Critical Assessment of Protein Structure Prediction conference, known as CASP14. This conference assesses how accurately different computational methods can predict protein structures. It has long been considered the benchmark for assessing these models against experimental methods.
At CASP14, AlphaFold vastly outperformed other computational methods and showed it could produce protein models that were accurate within the width of one atom. This is on par with experimental techniques.7
Entire PhD theses were once dedicated to solving a single protein’s structure. Now, AlphaFold could predict the structure in a matter of hours, or even minutes. It does this through a unique computational approach called deep learning.
The algorithm behind the AI
Taking inspiration from the complex inner workings of the brain, ‘deep learning’ uses artificial neural networks to process huge amounts of data. AlphaFold works by starting with an amino acid sequence, the string of beads which forms three-dimensional shapes. It also factors in known information about other proteins, as families of proteins with similar functions tend to have conserved sections that appear similar.
The AI program then searches through various databases for similar sequences, and collects relevant information about the physical, geometric, and evolutionary properties of these proteins. AlphaFold integrates all this data into its algorithm to assemble a preliminary three-dimensional protein structure. This process is iterated several times over, and with each iteration improving upon the previous prediction. Ultimately, this allows AlphaFold to output highly accurate protein structures.2,8
With this kind of technology at people’s fingertips, a wave of new scientific discoveries has been unleashed.
Invaluable impact
Access to AlphaFold has catalysed novel developments across science, technology, engineering, and mathematics (STEM), in areas like drug discovery, plastic pollution, and antibiotic resistance.
As one example, the AI program has been used to solve protein structures crucial to treating malaria. The structure of the protein Pfs48/45, which is involved in the development of the malaria parasite, was solved with the help of AlphaFold after many years of inconclusive experimentation by teams of researchers.9 A vaccine has been developed based on this protein, which has already successfully completed a phase I clinical trial as of 2022.10
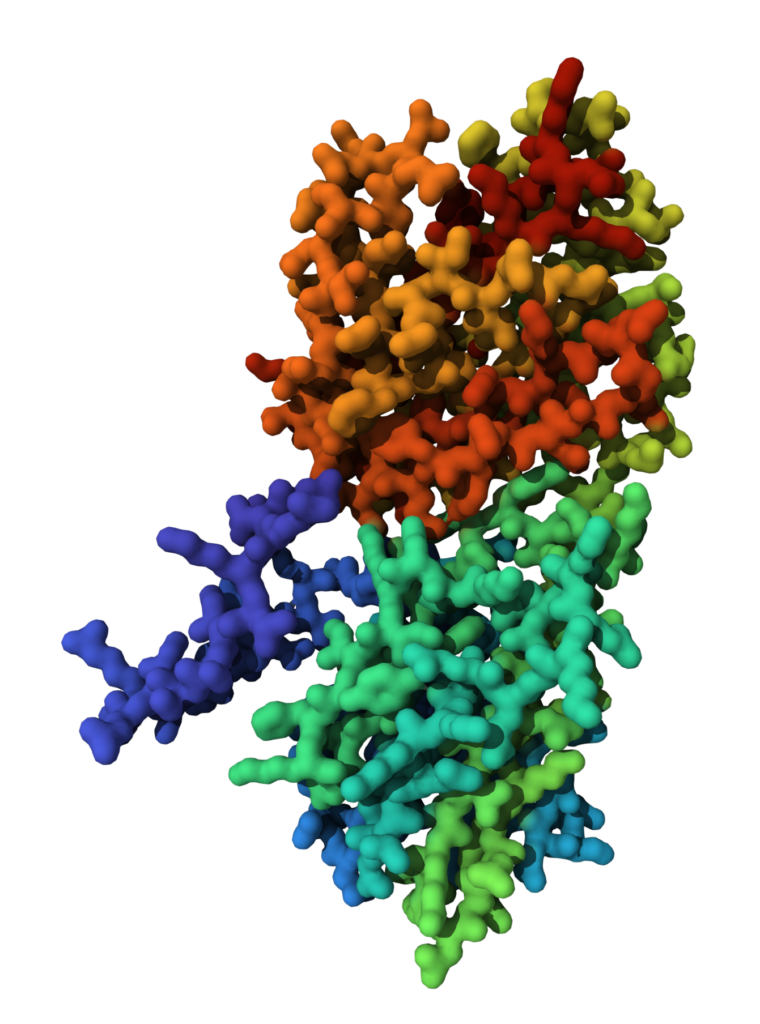
Proteins called enzymes serve a key role in countless biological and industrial processes, including breaking down plastic. Google DeepMind has partnered with the Centre for Enzyme Innovation at the University of Portsmouth to establish the protein structures of over 100 enzymes that could help break down chemicals in plastics, using AlphaFold. With this database, they aim to design enzymes that are cheaper, more structurally stable, and are faster acting to enhance plastic recycling.11
Antibiotic resistance is a growing healthcare crisis, and threatens our ability to effectively and safely treat a wide range of bacterial infections. The mechanism of resistance is often a bacterial protein that allows the organism to survive the drug, such as proteins that “pump” antibiotics out of the bacteria before they can be killed. One protein structure involved in a mechanism that causes bacterial resistance had evaded scientists for a decade. AlphaFold solved the protein structure in 30 minutes.12
This has accelerated research in this area and opened new possibilities for preventing deadly bacterial infections.13
A New Framework for the Future
For the most part, AlphaFold has solved the “protein folding problem”. It has accelerated scientific discovery and propelled research ideas into reality, where vaccines are entering the clinic and enzymes are being created to potentially conserve the environment. The developers of the AI program have also compiled the AlphaFold Protein Structure Database, which now includes over 200 million predicted protein structures, compared to the previous 200,000 in the PDB.14
As for the future of AlphaFold, it has already expanded into other versions designed for protein interactions or mutations. Although the AI program is highly accurate, it is still best used alongside experimental methods. This helps ensure that the protein structures it predicts really do transfer into the real world. Nevertheless, AlphaFold is being continually pushed to grow – competitors like RoseTTAFold, developed by the other Nobel Prize winner Professor Baker, are catching up quickly.15 With this ongoing progress, we are heading into an exciting future of important discoveries.
Brea Galea is a Master of Biomedical Science Student at Austin Health, The Florey, and The University of Melbourne.
References:
- Dill K. A., et al. (2008). The protein folding problem. Annu Rev Biophys, 37, 289-316. doi.org/10.1146/annurev.biophys.37.092707.153558
- Jumper J., et al. (2021). Highly accurate protein structure prediction with AlphaFold. Nature, 596, 583–589. doi.org/10.1038/s41586-021-03819-2
- Nobel Prize Outreach AB 2025. (2025). Press release. NobelPrize.org. www.nobelprize.org/prizes/chemistry/2024/press-release/
- Wrapp D., et al. (2020). Cryo-EM structure of the 2019-nCoV spike in the prefusion conformation. Science, 367(6483), 1260-1263. doi.org/10.1126/science.abb2507
- Xia S., et al. (2020). Inhibition of SARS-CoV-2 (previously 2019-nCoV) infection by a highly potent pan-coronavirus fusion inhibitor targeting its spike protein that harbors a high capacity to mediate membrane fusion. Cell Res, 30(4), 343-355. doi.org/10.1038/s41422-020-0305-x
- Berman H.M., et al. (2000). The Protein Data Bank. Nucleic Acids Research, 28, 235-242. doi.org/10.1093/nar/28.1.235
- Jumper J., et al. (2021). Applying and improving AlphaFold at CASP14. Proteins. 89(12), 1711-1721. doi.org/10.1002/prot.26257
- Howard Hughes Medical Institute. [HHMI’s Janelia Research Campus]. (2022). John Jumper: “Structure Prediction with AlphaFold”. [YouTube Video]. YouTube. www.youtube.com/watch?v=p1qjgkqwTdg
- Google DeepMind. (2022). Stopping malaria in its tracks. deepmind.google/discover/blog/stopping-malaria-in-its-tracks/
- Alkema M., et al. (2024). A Pfs48/45-based vaccine to block Plasmodium falciparum transmission: phase 1, open-label, clinical trial. BMC Med, 22, 170. doi.org/10.1186/s12916-024-03379-y
- University of Portsmouth. (2021). Enzyme researchers partner with pioneering AI company DeepMind. www.port.ac.uk/news-events-and-blogs/news/enzyme-researchers-partner-with-pioneering-ai-company-deepmind
- Google DeepMind. (2022). Accelerating the race against antibiotic resistance. deepmind.google/discover/blog/accelerating-the-race-against-antibiotic-resistance/
- Mitchell M. E., et al. (2023). Targeting the Conformational Change in ArnA Dehydrogenase for Selective Inhibition of Polymyxin Resistance. Biochemistry. 62(14), 2216-2227. doi.org/10.1021/acs.biochem.3c00227
- Varadi M., et al. (2022). AlphaFold Protein Structure Database: massively expanding the structural coverage of protein-sequence space with high-accuracy models. Nucleic Acids Res. 50(D1), D439-D444. doi.org/10.1093/nar/gkab1061
- Baek M., et al. (2024). Accurate prediction of protein-nucleic acid complexes using RoseTTAFoldNA. Nat Methods, 21(1), 117-121. doi.org/10.1038/s41592-023-02086-5